Can AI Solve Africa’s Energy Crisis? What You Need to Know
Introduction
Africa stands at a crossroads when it comes to energy. On the one hand, the continent boasts tremendous renewable energy potential—from its abundant sunshine to powerful wind corridors and vast hydropower resources. On the other hand, the energy crisis is very real: over 600 million people in sub-Saharan Africa live without reliable electricity, impacting everything from healthcare and education to economic development and quality of life. Meanwhile, the global drive for clean energy and carbon reduction places African nations in a unique position to leapfrog outdated grid systems.
But can artificial intelligence (AI) really transform this scenario? Can machine learning models, predictive analytics, and automated decision-making tools address the complex, structural challenges of Africa’s energy landscape?
That’s precisely what this long-form blog post will explore in-depth. We’ll shed light on:
- How AI-driven solutions can optimize renewable energy sources, grid management, and distribution networks across the continent.
- Real-world examples and case studies that illustrate the successes—and shortcomings—of AI applications in Africa’s power sector.
- A step-by-step blueprint for organizations looking to harness AI to tackle the continent’s energy shortage effectively.
- Common pitfalls to avoid, plus future insights that can help stakeholders remain agile in a rapidly evolving energy market.
- Actionable takeaways for governments, NGOs, startups, and established businesses aiming to make a difference—while also reaping significant ROI.
By the time you’ve finished reading, you’ll have a clearer understanding of how AI can be a game-changer for Africa’s energy crisis—and most importantly, how you or your organization can be part of the solution.
Table of Contents
- Understanding Africa’s Energy Landscape
- How AI Fits Into the Picture
- Core Applications of AI in Africa’s Energy Sector
- 3.1 Smart Grid Management
- 3.2 Renewable Energy Forecasting
- 3.3 Predictive Maintenance
- 3.4 Demand-Side Management
- 3.5 AI-Driven Off-Grid and Microgrid Solutions
- Step-by-Step Blueprint: Implementing AI Solutions for Energy Projects in Africa
- 4.1 Assessment and Feasibility Study
- 4.2 Technology Selection and Partnerships
- 4.3 Data Collection and Analysis
- 4.4 Pilot Testing and Scaling Up
- 4.5 Monitoring, Maintenance, and Ongoing Optimization
- Case Studies and Real-Life Examples
- 5.1 Mini-Grid Analytics in East Africa
- 5.2 Solar Power Forecasting in Morocco
- 5.3 Predictive Maintenance for Hydropower in Ethiopia
- Common Pitfalls and How to Avoid Them
- Future Insights/Trends
- 7.1 AI, Blockchain, and Carbon Credits
- 7.2 Resilient Grids for Climate Change
- 7.3 AI-Driven Financial Models
- Conclusion
- FAQs
1. Understanding Africa’s Energy Landscape
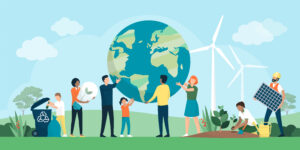
1.1 The Scope of the Crisis
Sub-Saharan Africa alone contains many of the fastest-growing economies in the world, yet the region consistently grapples with electricity access challenges. According to the International Energy Agency (IEA), over 600 million people do not have reliable access to electricity. This shortfall makes it extremely difficult to power schools, hospitals, and local businesses. When outages occur—or there’s no electricity at all—critical life-saving devices can’t run, online education halts, and economic productivity plummets.
1.2 Infrastructure Limitations
A significant barrier is the age and inefficiency of existing grid infrastructure. Much of it was built decades ago for populations a fraction of the size. Rapid urbanization compounds the problem, as city grids simply can’t handle the enormous influx of new residents. In rural areas, the costs of extending traditional grid lines are prohibitively high, making it difficult for grid operators and governments to justify such massive investment for relatively sparse populations.
1.3 A Renewables-First Approach
Interestingly, Africa has some of the world’s richest renewable energy resources—from the Sahara’s solar potential to the strong coastal winds around the continent. Harnessing these resources could help bridge the energy gap sustainably. Still, renewables—especially off-grid solar—come with variability: sunshine and wind are neither constant nor perfectly predictable. This is where intelligent forecasting and advanced data analytics become essential.
1.4 The Role of AI
So, why is AI such a big deal for Africa’s energy crisis? One simple word: optimization. AI can help:
- Forecast Demand: Predict how much power is needed in specific regions at specific times.
- Manage Grids: Distribute available power in the most efficient manner.
- Automate Off-Grid Systems: Deploy microgrids or mini-grids capable of self-regulating supply and demand.
By leveraging machine learning, deep learning, and predictive analytics, African nations can do more with less—an approach that’s crucial in regions where budgets are tight, and resource constraints are the norm.
2. How AI Fits Into the Picture
2.1 Data-Driven Decision Making
Artificial intelligence thrives on quality data. With the rise of IoT sensors and smart meters, energy providers can now collect real-time data on energy consumption, grid performance, and even consumer behavior. This data, when fed into AI algorithms, translates into actionable insights for everything from load balancing to maintenance scheduling. In an environment where every kilowatt-hour is precious, these insights are critical to eliminate waste and ensure equitable distribution.
2.2 Speed and Scalability
The complexity of balancing multiple energy sources—solar, wind, hydro, and even diesel generators in some regions—exceeds the capacity of human analysts working manually. AI systems can sift through massive data sets almost instantly, detecting anomalies, predicting failures, and recommending the most cost-effective operational strategies. As African countries continue to scale up or upgrade their grids, automation becomes indispensable.
2.3 AI Democratizing Energy Access
AI also enables micro-level solutions. Consider a small village with a solar-powered microgrid. AI algorithms can determine which homes or devices get priority if supply is low, or how best to store energy in batteries during peak sunlight. The entire system can function autonomously, with minimal external oversight. This democratizes energy access by making off-grid setups more self-sufficient and resilient, even in remote communities.
2.4 Barriers to Adoption
However, challenges remain:
- High Upfront Costs: Installing sensors, AI software, and advanced equipment can be expensive.
- Technical Skills Gap: Africa needs more AI and data science experts, especially those familiar with energy systems.
- Policy and Regulatory Issues: Many nations lack the legal frameworks to integrate and govern these new technologies effectively.
Despite these hurdles, the long-term benefits—improved energy reliability, cost savings, and environmental sustainability—make AI an enticing path forward.
3. Core Applications of AI in Africa’s Energy Sector
3.1 Smart Grid Management
Smart grids are electricity networks that use digital communications to detect and react to local changes in usage. When you add AI into the mix, these grids become even smarter. AI-driven solutions can:
- Monitor Grid Health: Identify faults or outages in near-real-time.
- Automate Load Balancing: Distribute available power efficiently to where it’s needed most.
- Adapt to Fluctuations: Adjust supply based on the intermittent nature of renewables.
Example: South Africa’s Grid Challenges
South Africa has long struggled with load shedding—rolling blackouts designed to prevent the grid from collapsing under stress. AI-driven solutions could help predict peak usage times and automatically redistribute load, significantly reducing these forced outages.
3.2 Renewable Energy Forecasting
Solar and wind energy are inherently variable. Cloud cover or changing wind speeds can drastically affect output, creating uncertainty for operators. AI-powered forecasting tools analyze historical data, satellite imagery, and weather models to predict short-term and long-term power generation more accurately. This helps grid managers plan ahead and minimize wastage or shortages.
Example: Wind Farms in Northern Kenya
Northern Kenya is ripe with strong winds, but unpredictable gusts have complicated the Lake Turkana Wind Power project. Machine learning models that incorporate local climate data could reduce the risk of downtime, ensuring more consistent energy supply to the Kenyan grid.
3.3 Predictive Maintenance
Equipment failures can be catastrophic, especially in regions where replacement parts and skilled technicians are limited. AI-driven predictive maintenance solutions collect sensor data on key parameters—like temperature, vibration, or output efficiency—to preemptively alert operators of potential breakdowns.
Benefit
- Reduced Downtime: Catching problems early prevents long outages.
- Lower Repair Costs: Maintenance can be planned, avoiding expensive emergency measures.
- Extended Lifespan: Proper upkeep prolongs the operational life of turbines, solar panels, or distribution transformers.
3.4 Demand-Side Management
Instead of focusing solely on supply, AI can also optimize demand. For instance, smart meters connected to AI algorithms can suggest times for households to use large appliances—like washing machines or electric cookers—to avoid peak load periods. Some systems even offer financial incentives for users who shift their usage to off-peak times.
Real-World Impact
In bustling city centers like Nairobi or Lagos, AI-driven demand-side management can help reduce stress on the grid, especially during periods of high economic activity (e.g., midday business hours). This, in turn, lowers energy prices and increases the reliability of supply for all.
3.5 AI-Driven Off-Grid and Microgrid Solutions
With much of rural Africa too remote for conventional grid connections, off-grid and microgrid setups are crucial. AI enables these systems to:
- Prioritize Critical Loads: Ensure essential services like clinics or schools remain powered.
- Match Energy Supply with Demand: Optimize battery storage usage to minimize waste.
- Automate Billing and Maintenance: Residents can receive real-time info about their usage and system performance via mobile apps.
In short, AI makes rural electrification more sustainable by streamlining operations, reducing costs, and enhancing community engagement.
4. Step-by-Step Blueprint: Implementing AI Solutions for Energy Projects in Africa
Successfully integrating AI into Africa’s energy sector requires careful planning and targeted execution. Below is a step-by-step blueprint designed for governments, NGOs, private sector players, and local communities.
4.1 Assessment and Feasibility Study
- Identify Energy Needs: Determine if the goal is to improve grid stability, expand off-grid access, or both.
- Set Clear KPIs: Define success metrics (e.g., reduction in downtime, improved forecasting accuracy, or number of new connections).
- Conduct Site Surveys: Gather data on infrastructure, local resources (sunlight, wind potential, rivers for hydro), and population energy consumption patterns.
Best Practice: Engage local communities early to understand cultural, economic, and logistical nuances that could affect project success.
4.2 Technology Selection and Partnerships
- Choose the Right AI Platform: Evaluate whether you need cloud-based analytics, on-premises solutions, or edge computing for real-time decisions in off-grid scenarios.
- Form Collaborations: Partner with universities, tech companies, or international donors with expertise in energy and AI.
- Hardware Considerations: Ensure all hardware (sensors, data loggers, solar inverters) is robust enough to withstand local climate conditions—e.g., extreme heat, dust, or humidity.
Pro Tip: Look into open-source AI tools (like TensorFlow or PyTorch) for cost-effective solutions.
4.3 Data Collection and Analysis
- IoT Integration: Install sensors or smart meters to capture real-time data on energy generation and usage.
- Data Pipeline Setup: Establish how data flows from sensors to your AI models—whether via cellular networks, satellites, or local Wi-Fi.
- Data Cleaning and Validation: Africa’s diverse conditions can lead to noise or incomplete datasets. Build robust data cleaning protocols to ensure reliable analytics.
Tool Recommendation: Platforms like Kibana and Grafana can help visualize real-time data streams for quick insights.
4.4 Pilot Testing and Scaling Up
- Initial Rollout: Start with a smaller pilot—maybe a single microgrid or a local substation.
- Monitoring: Track performance metrics daily or weekly. Use AI dashboards to identify bottlenecks.
- Feedback Loop: Gather input from technicians, community members, and local partners. Make iterative improvements.
- Scale: Once proven viable, expand regionally or nationally. Seek additional funding if needed from government grants or international investors.
Insight: Pilots often expose unforeseen challenges like specialized maintenance requirements or local regulatory barriers, so remain flexible in your approach.
4.5 Monitoring, Maintenance, and Ongoing Optimization
- Regular Audits: Conduct both digital and physical inspections of hardware and software.
- Continuous Learning: Update AI models with new data to refine predictions and optimization routines.
- Local Capacity Building: Train local operators and technicians to manage and troubleshoot AI systems, fostering self-reliance.
Why It Matters: Sustained success hinges on local ownership and the ability to adapt as conditions change, from population growth to shifting climate patterns.
5. Case Studies and Real-Life Examples
5.1 Mini-Grid Analytics in East Africa
Context: An East African NGO collaborated with a local tech company to install AI-enhanced mini-grids in rural Tanzania.
- Challenge: Frequent disruptions due to inaccurate load estimates and over-dependence on diesel backups.
- AI Solution: They deployed machine learning models to analyze historical usage patterns, forecast weather impacts on solar output, and automate battery charging schedules.
- Outcome: The system cut diesel generator usage by 40%, saving funds and reducing carbon emissions. Local businesses reported more consistent power, allowing for extended hours of operation and improved community well-being.
5.2 Solar Power Forecasting in Morocco
Context: Morocco has aggressively invested in solar farms, notably the Noor solar complex near Ouarzazate. However, solar output can fluctuate significantly with seasonal sandstorms and cloud cover.
- Challenge: The national grid operator struggled with short-term forecasting inaccuracies, leading to frequent over-reliance on fossil fuels for backup.
- AI Solution: A partnership with a European AI firm introduced satellite-based cloud prediction algorithms. Combining these with on-ground sensors drastically improved forecast accuracy.
- Outcome: The grid operator was able to more confidently schedule hydro and thermal power plants, leading to efficient load balancing and significant cost savings.
5.3 Predictive Maintenance for Hydropower in Ethiopia
Context: Ethiopia relies heavily on hydropower from the Blue Nile and its tributaries.
- Challenge: Corrosion, debris buildup, and mechanical wear often led to unexpected plant shutdowns.
- AI Solution: Engineers installed sensors to monitor water flow rates, turbine vibration levels, and temperature. Predictive models flagged potential issues days or weeks in advance.
- Outcome: Scheduled maintenance replaced emergency repairs, reducing downtime by an estimated 25% and boosting overall energy production.
Each of these examples underscores how AI isn’t a one-size-fits-all approach; rather, it’s a tool that, when adapted to local conditions, can yield transformational results in Africa’s energy sector.
6. Common Pitfalls and How to Avoid Them
- Over-Reliance on Imported Tech
- Issue: Fully imported solutions might not account for Africa’s unique grid conditions, climatic extremes, or local labor skills.
- Solution: Engage local developers and technicians early. Encourage knowledge transfer and customization to regional realities.
- Neglecting the Human Factor
- Issue: Focusing on technical brilliance without considering social, cultural, and economic aspects can lead to underutilized or misused systems.
- Solution: Involve community stakeholders, provide adequate training, and consider affordability in design.
- Data Quality Issues
- Issue: AI models can be severely compromised by incomplete or inaccurate data, especially in remote or conflict-prone areas.
- Solution: Build robust data validation and cleaning processes. Use multiple data sources (e.g., satellite imagery, on-site sensors, user surveys) to cross-check results.
- Unclear ROI
- Issue: Funding is tight, and if an AI project doesn’t clearly demonstrate return on investment, it may stall.
- Solution: Align AI objectives with bottom-line benefits—lower operational costs, improved reliability, or revenue from new user connections.
- Lack of Policy Support
- Issue: Governments sometimes lack the frameworks needed to incorporate AI solutions seamlessly.
- Solution: Collaborate with policymakers, show them the socio-economic benefits of AI adoption, and advocate for clearer guidelines and incentives.
Avoiding these pitfalls requires strategic planning, local partnerships, and continuous evaluation. Think of AI not as a cure-all, but as a powerful ally in addressing the region’s complex challenges.
7. Future Insights/Trends
7.1 AI, Blockchain, and Carbon Credits
Trend: We’re starting to see the convergence of blockchain with AI to monitor and verify carbon offset activities, especially relevant for African nations hosting reforestation or green energy projects. With smart contracts, local communities can earn carbon credits for preserving forests or running solar farms, all tracked via tamper-proof ledgers. AI then validates these offsets, ensuring transparency and accountability.
What This Means: Innovative financing models could emerge, allowing African energy projects to tap into global carbon markets for additional revenue.
7.2 Resilient Grids for Climate Change
Trend: As global warming intensifies, extreme weather events become more frequent, threatening electricity infrastructure. AI-powered resilience planning can help grids anticipate storms, floods, or droughts, enabling more targeted pre-emptive measures.
What This Means: Governments and donors will increasingly fund AI solutions that bolster climate resilience—reinforcing or retrofitting existing systems to handle extreme conditions.
7.3 AI-Driven Financial Models
Trend: Financial institutions are starting to use AI to evaluate creditworthiness for energy ventures, including individual home solar installations. Instead of solely relying on traditional banking metrics, algorithms can analyze local economic activity, weather patterns, and community uptake rates, making off-grid financing more accessible.
What This Means: Greater inclusion for rural and underbanked populations, as data-driven risk assessments lower interest rates and collateral requirements.
8. Conclusion
So, can AI solve Africa’s energy crisis? While no single technology can magically erase decades of underinvestment and infrastructural challenges, AI stands out as a transformational tool. Its capabilities in forecasting, predictive maintenance, demand-side management, and smart grid operations can radically enhance reliability, reduce costs, and expand access—especially when coupled with local expertise and robust policy backing.
Key Takeaways:
- AI Is an Enabler: It’s not a silver bullet but can maximize the impact of renewable energy investments.
- Adaptation Is Essential: Solutions must be culturally, environmentally, and economically tailored.
- Collaboration: Partnerships between governments, tech firms, NGOs, and local communities are vital for project success.
- Long-Term Perspective: Think in terms of scalability and sustainability—not quick fixes.
Call to Action
Are you inspired to be part of the AI-driven energy revolution in Africa?
- Download Our In-Depth Checklist for implementing AI in emerging market energy projects—perfect for NGOs, startups, and government agencies.
- Subscribe to Our Newsletter to stay updated on the latest breakthroughs, funding opportunities, and success stories in AI and renewable energy across the continent.
- Schedule a Consultation with our team of experts to discuss how you can optimize your energy initiatives, secure grants, or build local partnerships to drive sustainable growth.
By taking these steps, you align yourself with a growing global movement determined to deliver cleaner, more reliable power to millions—fueling education, healthcare, entrepreneurship, and ultimately, a brighter future for all Africans.
Read Also: Top 7 Machine Learning Models Used in Clean Energy Forecasting
9. FAQs
1. Is AI too expensive for smaller African communities or NGOs?
AI implementation can be more cost-effective than you might think, especially when using open-source software and basic hardware. Small-scale pilot projects often tap into grant funding or philanthropic support to offset initial costs. Plus, long-term savings from optimized operations and reduced downtime can quickly recoup the investment.
2. Which African countries are currently leading in AI for energy?
Countries like Kenya, South Africa, Morocco, and Nigeria are among the frontrunners, thanks to proactive government policies and robust tech ecosystems. That said, smaller nations like Rwanda and Botswana also have rising AI initiatives, often supported by international donors.
3. Can AI work in off-grid or rural settings without stable internet?
Yes. Edge computing solutions allow AI models to run on local hardware without continuous internet access. Some systems also store data offline and upload it periodically when connectivity is available. This ensures communities remain autonomous in managing their energy systems.
4. What about the risk of job displacement?
While automation could streamline certain tasks, AI in the energy sector often creates new skilled job opportunities—from data analysts to tech support roles. The focus is on upskilling the local workforce so that they can manage, maintain, and even innovate new AI solutions.
5. How can governments support AI adoption for energy?
Governments can offer financial incentives, develop favorable regulations, and invest in capacity-building programs. Collaborations with universities and research institutes can also cultivate local AI talent, ensuring homegrown solutions that understand regional needs.